Trending Insights
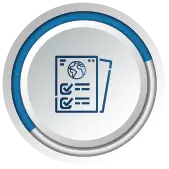
Global Leaders in Strategy and Innovation Rely on Our Expertise to Seize Growth Opportunities
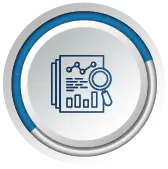
Our Research is the Cornerstone of 1000 Firms to Stay in the Lead
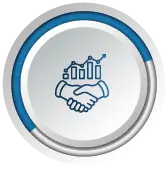
1000 Top Companies Partner with Us to Explore Fresh Revenue Channels
US Tariff Impact on Data Annotation Market
Trump Tariffs Ignite Global Business Evolution
Request FREE sample PDF 
Pharmacy benefit management market
DATA ANNOTATION MARKET OVERVIEW
The global Data Annotation Market was valued at USD 2.87 Billion in 2024 and is expected to grow to USD 23.82 Billion by 2033, with a projected CAGR of 26.5% during the forecast period 2024 to 2033.
The Data Annotation Market plays a crucial role in the development of artificial intelligence (AI) and machine learning (ML) models, providing accurately labeled datasets that enable algorithms to recognize patterns and make intelligent decisions. As AI adoption grows across industries such as healthcare, automotive, finance, and IT, the need for high-quality annotated data has skyrocketed. Data annotation involves categorizing, tagging, and labeling text, images, videos, and audio files to train AI models effectively. Businesses and research institutions rely on both automated and human-in-the-loop annotation methods to enhance AI accuracy.
The increasing demand for autonomous systems, natural language processing (NLP), and computer vision applications has further accelerated market growth. Companies are investing in advanced annotation tools powered by AI-assisted labeling and crowdsourced human annotators to improve data accuracy and efficiency. However, challenges such as data privacy concerns, high annotation costs, and maintaining annotation consistency persist. Despite these obstacles, the market is expected to grow significantly, driven by the expansion of AI-driven industries and continuous advancements in annotation technology. As AI models become more sophisticated, the Data Annotation Market will remain a critical enabler of innovation and automation.
COVID-19 IMPACT
"Data Annotation Market Industry Had a positive impact due to during COVID-19 Pandemic"
The global COVID-19 pandemic has been unprecedented and staggering, with the market experiencing Higher-than-anticipated demand across all regions compared to pre-pandemic levels. The sudden market decline reflected by the rise in CAGR is attributable to the market’s decline and demand returning to pre-pandemic levels.
The COVID-19 pandemic initially caused disruptions in the Data Annotation Market due to workforce limitations, delays in annotation projects, and reduced investments in AI development. Many data labeling companies relied on human annotators, often working in large teams, and lockdowns led to operational challenges and slowed project timelines. Additionally, budget constraints caused by economic uncertainty forced some companies to delay AI implementation, impacting short-term demand for annotation services.
However, as businesses adapted to remote work and digital transformation accelerated, the Data Annotation Market saw a significant rebound. The pandemic heightened reliance on AI-driven solutions in healthcare, e-commerce, and automation, driving demand for high-quality labeled data. Industries such as telemedicine, contactless services, and supply chain automation required improved AI models, leading to increased investments in data annotation. Companies also shifted towards AI-assisted annotation tools to reduce dependency on human labelers and ensure scalability.
Ultimately, while COVID-19 caused temporary disruptions in the market, it had an overall positive long-term impact by emphasizing the importance of AI-powered automation. The post-pandemic world continues to witness sustained growth in AI adoption, ensuring a strong and expanding market for data annotation services.
LATEST TREND
The Data Annotation Market is evolving rapidly, driven by technological advancements and the growing need for high-quality training data in AI development. One of the most significant trends shaping the industry is the rise of AI-assisted annotation tools that use machine learning to automate and accelerate the labeling process. These tools leverage pre-trained models to suggest annotations, significantly reducing the manual workload for human labelers. AI-assisted annotation enhances efficiency while maintaining accuracy, making it a preferred choice for large-scale data labeling projects. Another emerging trend is the increasing demand for video annotation due to the rise of computer vision applications in sectors like autonomous driving, security, and augmented reality. Video annotation requires frame-by-frame labeling of objects, actions, and movements, making it more complex than static image annotation. Companies are investing in advanced video labeling solutions to improve AI-driven surveillance, automated navigation, and behavioral analysis models. Additionally, crowdsourced data annotation platforms are gaining traction, allowing businesses to scale their annotation tasks by distributing work among a global workforce. This method enhances efficiency and provides diverse datasets essential for training unbiased AI models. Among these trends, AI-assisted annotation stands out as the most transformative, as it combines human expertise with automation to improve speed and accuracy. As AI models continue to require vast amounts of annotated data, these innovations will play a crucial role in shaping the future of the Data Annotation Market.
DATA ANNOTATION MARKET SEGMENTATION
By Type
Based on Type, the global market can be categorized into
- Text Annotation Text annotation involves labeling words, sentences, or phrases to train AI models in natural language processing (NLP). It includes tasks like named entity recognition, sentiment analysis, and part-of-speech tagging. Text annotation is essential for developing AI-driven chatbots, virtual assistants, and search engines. Businesses in e-commerce and social media leverage annotated text data for personalized recommendations. The increasing adoption of AI in content moderation and language translation is driving demand for text annotation services.
- Image/Video Annotation Image and video annotation involve labeling objects, faces, or gestures to improve computer vision applications. Autonomous vehicles rely on image annotation to detect pedestrians, traffic signs, and lane boundaries. In healthcare, annotated medical images assist AI models in diagnosing diseases with higher accuracy. Security and surveillance systems use video annotation to enhance facial recognition and object tracking. The growing demand for AI-powered visual recognition tools is propelling the expansion of this segment.
- Audio Annotation Audio annotation involves transcribing and labeling sound recordings for AI-powered speech recognition systems. It plays a crucial role in developing voice assistants, automated transcription services, and speech-to-text applications. Audio annotation includes tasks like speaker diarization, emotion detection, and language identification. Industries such as customer service, media, and accessibility solutions heavily rely on annotated audio datasets. With the rise of voice-controlled devices and smart assistants, the demand for high-quality annotated audio data continues to grow.
By Application
Based on application, the global market can be categorized
- IT The IT industry heavily relies on data annotation for developing AI-powered chatbots, automated coding assistants, and cybersecurity solutions. Labeled text and image data help AI models detect threats, automate processes, and enhance user experiences. Tech companies use annotated datasets to improve search algorithms and recommendation systems. AI-driven content moderation tools in social media and online platforms depend on accurate data labeling. The continuous evolution of AI applications ensures steady demand for data annotation services in IT.
- Automotive The automotive industry requires extensive data annotation for developing self-driving cars and advanced driver-assistance systems (ADAS). Annotated image and video data help AI models recognize road signs, pedestrians, and lane markings. LiDAR and sensor-based annotation are essential for enhancing vehicle perception in real-world driving conditions. Automakers collaborate with data annotation companies to refine AI-powered navigation and obstacle detection. The push toward autonomous mobility is accelerating growth in this segment.
- Government Governments use data annotation for surveillance, law enforcement, and defense AI applications. Annotated video and image datasets enhance facial recognition and object detection in security systems. AI-driven data processing helps in automating administrative tasks and fraud detection. Governments also utilize annotated text data for policy analysis and automated document classification. Increased investment in AI-driven public sector initiatives fuels demand for data annotation services.
- Healthcare The healthcare industry leverages data annotation for AI-assisted diagnostics, medical imaging, and drug discovery. Annotated medical images help train AI models to detect diseases like cancer, fractures, and neurological disorders. Speech annotation aids in developing AI-driven patient interaction tools. Labeled healthcare data enhances predictive analytics for personalized treatment plans. The growing role of AI in medical research and diagnostics is driving demand for healthcare-focused data annotation.
- Financial Services Financial institutions use data annotation for fraud detection, risk assessment, and AI-driven customer support. Annotated text data enhances chatbots and automated financial advisory services. Image and document annotation help AI models process identity verification and compliance-related tasks. AI-driven sentiment analysis in financial markets relies on labeled datasets. The increasing adoption of AI in fintech solutions is expanding the need for precise financial data annotation.
MARKET DYNAMICS
Market dynamics include driving and restraining factors, opportunities and challenges stating the market conditions.
Drivng Factor
"Rising Demand for AI and Machine Learning Applications"
The increasing adoption of artificial intelligence (AI) and machine learning (ML) across industries is a major driver of the data annotation market. AI models require vast amounts of accurately labeled data to improve their learning algorithms, making data annotation a crucial component in AI development. From healthcare and autonomous vehicles to e-commerce and finance, organizations are investing heavily in annotated datasets to enhance AI-powered applications. As AI adoption expands across industries, the need for high-quality data labeling continues to grow, driving market demand.
"Growth of Computer Vision and Natural Language Processing (NLP)"
Advancements in computer vision and natural language processing (NLP) have accelerated the demand for precisely annotated data. Applications such as facial recognition, object detection, and automated content moderation rely on labeled images and videos for accuracy. Similarly, chatbots, voice assistants, and sentiment analysis tools require annotated text data to understand human language effectively. As businesses integrate these AI-driven technologies into their operations, the demand for data annotation services is expected to surge, fostering market growth.
Restraining Factor
One of the primary restraining factors in the data annotation market is the high cost and time-consuming nature of manual annotation. Data labeling is a labor-intensive process that requires human expertise, leading to high operational expenses for companies that rely on annotated datasets. The need for meticulous accuracy further increases costs, as errors in data labeling can significantly impact AI model performance.
Moreover, manual annotation is time-consuming, especially for large datasets used in industries such as autonomous driving, healthcare, and retail. Companies must allocate substantial resources to maintain workforce efficiency and quality control, making scalability a challenge. While automation has improved annotation efficiency, complex tasks like sentiment analysis, medical imaging, and video annotation still require human intervention.
Another issue is the inconsistency in data labeling quality. Since different annotators may interpret data differently, ensuring uniformity in labeling becomes difficult, leading to biased or inaccurate datasets. This inconsistency can affect the reliability of AI models, forcing businesses to invest additional time and resources in refining their datasets.
Additionally, privacy and security concerns related to handling sensitive data, such as medical records and financial transactions, pose another limitation. Strict data protection regulations make it challenging for companies to outsource annotation services, restricting market expansion in highly regulated industries.
Opportunity
"Increasing adoption of automated data annotation tools powered by AI"
A significant opportunity in the data annotation market lies in the increasing adoption of automated data annotation tools powered by AI. As businesses strive to enhance efficiency and reduce costs, automation in data labeling is emerging as a transformative solution. AI-powered annotation tools leverage machine learning algorithms to pre-label data, significantly reducing the time and labor required for manual annotation.
Industries such as healthcare, autonomous vehicles, and e-commerce are adopting AI-driven annotation techniques to streamline their data labeling processes. For instance, in medical imaging, AI-powered tools can automatically detect and annotate abnormalities in X-rays or MRIs, assisting radiologists in diagnosis. Similarly, in the automotive sector, autonomous vehicle manufacturers are integrating AI-based annotation solutions to speed up the labeling of sensor data.
Another area of opportunity is the integration of crowdsourcing platforms for scalable data annotation. Companies are increasingly utilizing global annotation workforces to scale operations cost-effectively. Platforms like Amazon Mechanical Turk and other data-labeling crowdsourcing solutions allow businesses to distribute annotation tasks across a vast pool of contributors, enhancing efficiency.
As AI models become more sophisticated and require increasingly complex datasets, the demand for hybrid annotation models (a combination of automated and human-assisted labeling) will continue to grow. Companies investing in automation and hybrid solutions stand to gain a competitive edge, fostering significant market expansion.
Challenge
"Ensuring high-quality and unbiased data labeling"
One of the most significant challenges in the data annotation market is ensuring high-quality and unbiased data labeling. The accuracy of AI and ML models heavily depends on the quality of labeled data, and any inconsistency or bias in annotation can lead to flawed AI predictions.
Bias in data annotation arises when human labelers introduce subjective or cultural interpretations while tagging data. For instance, facial recognition AI systems have faced criticism for racial and gender biases due to imbalanced or inaccurately labeled training datasets. Similarly, sentiment analysis models may misinterpret sarcasm or context due to inconsistent annotation in text-based data. These biases negatively impact AI decision-making and create ethical concerns, especially in areas like recruitment, law enforcement, and financial services.
Ensuring consistency across large-scale datasets is another major challenge. Different annotators may label similar data points differently, leading to discrepancies in model training. This is particularly problematic in complex tasks like medical data annotation, where mislabeling can have severe consequences.
To address these challenges, companies must implement strict quality control measures, such as multiple annotation layers, cross-validation techniques, and AI-assisted validation tools. Additionally, increasing diversity in annotation workforces and training annotators to recognize and mitigate bias is essential. However, these measures require additional resources, adding to operational costs and making scalability difficult.
As AI adoption continues to grow, the industry must find innovative solutions to minimize bias and ensure consistency in data labeling, making it a crucial challenge for the future of the data annotation market.
DATA ANNOTATION MARKET REGIONAL INSIGHTS
-
North America
North America leads the data annotation market due to the strong presence of AI-driven companies, advanced technological infrastructure, and significant investments in machine learning research. The region is home to tech giants such as Google, Amazon, and Microsoft, all of which rely on high-quality annotated data to train AI models. Additionally, government initiatives supporting AI development have fueled the demand for data labeling services. The United States Data Annotation Market plays a pivotal role in North America's dominance, driven by the rapid expansion of AI applications in healthcare, autonomous vehicles, and e-commerce. Major U.S.-based AI companies are investing heavily in annotation technologies, further strengthening market growth.
-
Europe
Europe is a key contributor to the data annotation market growth, driven by stringent AI regulations, strong research initiatives, and a growing focus on ethical AI development. Countries like Germany, France, and the UK are investing in high-quality data labeling to support AI advancements across industries. The European Union’s emphasis on GDPR compliance has also created demand for secure and privacy-focused annotation solutions. Additionally, the region’s robust automotive and healthcare sectors rely on accurate data annotation for autonomous vehicles and medical AI applications.
-
Asia
Asia is rapidly emerging as a major player in the data annotation market due to low-cost labor, increasing AI adoption, and the presence of leading AI research centers. Countries like China, India, and Japan have seen a surge in AI-driven businesses, fueling the need for large-scale data labeling. India, in particular, is a hub for outsourced annotation services due to its cost-effectiveness and skilled workforce. Meanwhile, China’s investments in AI and smart city projects are driving demand for high-quality labeled datasets. The growing digital economy and AI research in Asia are expected to accelerate market growth.
KEY INDUSTRY PLAYERS
Key industry players in the data annotation market significantly shape its growth through technological innovation, automation, and global expansion strategies. Companies such as Playment, Hive, and Lotus Quality Assurance are pioneering AI-powered annotation tools that enhance labeling efficiency and accuracy. These firms are integrating machine learning into their annotation processes to reduce manual workload and improve scalability.
Additionally, major players invest in quality control mechanisms to ensure high-quality labeled datasets. Techniques such as multi-layer validation, consensus-based labeling, and AI-assisted error detection are being implemented to address annotation inconsistencies. This enhances the reliability of AI models trained on their datasets.
The global expansion of annotation services has also played a key role in market growth. Leading companies have set up offshore annotation centers in regions with cost-effective labor, such as India and Southeast Asia, to scale operations while maintaining affordability.
Moreover, industry leaders are forging strategic partnerships with AI developers, cloud service providers, and government organizations to expand their market presence. Their investments in automation, ethical AI, and scalable solutions will continue to drive the data annotation industry forward.
List of Top Data Annotation Companies
- Playment Inc – India
- Explosion – Germany
- Lotus Quality Assurance – Vietnam
- Tagtog – Belgium
- Hive – United States
KEY INDUSTRY DEVELOPMENT
"Appen’s Acquisition of Quadrant – March 2023"
In March 2023, Appen, a global leader in AI data annotation, acquired Quadrant, a geolocation data and technology company. This strategic acquisition aims to enhance Appen’s data labeling capabilities by integrating location-based intelligence into its AI training datasets. The move strengthens Appen’s ability to provide high-quality annotated data for applications in navigation, autonomous vehicles, and AI-driven location services. By expanding its dataset offerings, Appen caters to the growing demand for more precise and context-aware AI models. This acquisition aligns with the broader industry trend of enhancing AI training data with real-world, high-accuracy geospatial annotations.
"Scale AI’s Partnership with the U.S. Department of Defense – October 2023"
In October 2023, Scale AI, a leading provider of data annotation services, secured a multi-million-dollar contract with the U.S. Department of Defense (DoD) to provide high-quality annotated data for military AI applications. The partnership focuses on improving computer vision models, autonomous defense systems, and AI-powered reconnaissance tools. Scale AI’s expertise in large-scale data labeling will help the military develop more advanced machine learning models with better object detection and classification capabilities. The collaboration highlights the increasing importance of accurate data annotation in defense and security applications. By working with the DoD, Scale AI strengthens its position as a key player in the high-stakes AI training industry.
REPORT COVERAGE
The study encompasses a comprehensive SWOT analysis and provides insights into future developments within the market. It examines various factors that contribute to the growth of the market, exploring a wide range of market categories and potential applications that may impact its trajectory in the coming years. The analysis takes into account both current trends and historical turning points, providing a holistic understanding of the market's components and identifying potential areas for growth.
This research report examines the segmentation of the market by using both quantitative and qualitative methods to provide a thorough analysis that also evaluates the influence of strategic and financial perspectives on the market. Additionally, the report's regional assessments consider the dominant supply and demand forces that impact market growth. The competitive landscape is detailed meticulously, including shares of significant market competitors. The report incorporates unconventional research techniques, methodologies and key strategies tailored for the anticipated frame of time. Overall, it offers valuable and comprehensive insights into the market dynamics professionally and understandably.
REPORT COVERAGE | DETAILS |
---|---|
Market Size Value In |
US$ 2.87 Billion in 2024 |
Market Size Value By |
US$ 23.82 Billion by 2033 |
Growth Rate |
CAGR of 26.5% from 2024 to 2033 |
Forecast Period |
2025-2033 |
Base Year |
2024 |
Historical Data Available |
yes |
Regional Scope |
global |
Segments Covered | |
By Type
|
|
By Application
|
Frequently Asked Questions
-
What value is the Data Annotation Market expected to touch by 2033?
The global Data Annotation Market is expected to reach approximately USD 23.82 Billion by 2033.
-
What CAGR is the Data Annotation Market expected to exhibit by 2033?
The Data Annotation Market is expected to exhibit a CAGR of 26.5% by 2033.
-
What are the key Data Annotation Market segments?
The key Data Annotation market segmentation, which includes, based on type Text Image/Video, Audio & By Application IT, Automotive, Government, Healthcare, Financial Services.
-
Which is the leading region in the Data Annotation Market?
U.S Pacific is the prime area for the Data Annotation Market owing to its high consumption and cultivation.
-
What are the driving factors of the Data Annotation Market?
Growth of Computer Vision and Natural Language Processing (NLP) and Rising Demand for AI and Machine Learning Applications are the driving factors in the Data Annotation market.